Data protection is a challenge for the security industry. It is prone to disastrous attacks to illegally access data, disrupt digital operations, or damage information. Moreover, the difficulty and vulnerability continue to rise with the arrival of web-connected devices. Adding fuel to the fire is the lack of skilled talent that fails to mitigate the expanding stress.
With this burgeoning disruption, a notable wing of technology development that’s helping to avert such security risks is machine learning. Yes, the emergence of machine learning for information security has opened a new world by harnessing the strength of data and automation.
Why is Machine Learning in Information Security (InfoSec) a Need?
Since InfoSec deals with all the digital and non-digital data, it often leads to data destruction, modification, and disruption. Security experts must account for the safeguard of data irrespective of what format and setup it is in. But, machine learning in information security can come as a saviour to tackle all these challenges with ease.
The following issues in the digital world call for correction and signal the need to implement machine learning in network security.
- Cyber Attacks: Today, hackers use undetected malware and agile methodologies to compromise and violate data – a problem that legacy systems cannot deal with.
- Weak Links in Businesses’ Systems: Traditionally, InfoSec was treated as an IT problem, and it isn’t entirely wrong. Attacks can crop up from any weak link in the organization irrespective of the department or hierarchy, so it is important to shield the entire organization by absolute and impeccable security programs.
- An Array of Threat Classification: The wide range of sources and causes of security breaches signals the need for machine learning algorithms for network security. Threats and cyber attacks are technical hardware failure issues, acts of espionage, or human errors. The InfoSec system needs to account for these and merge with AI and ML to tackle these security threats.
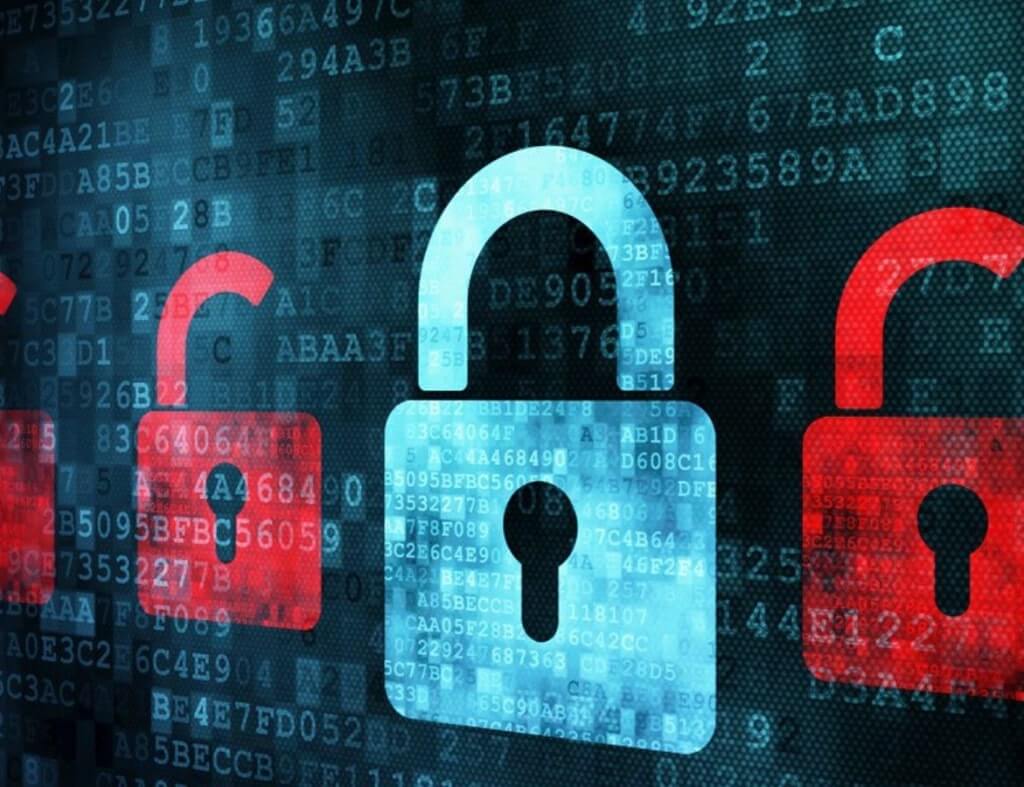
How Can Machine Learning for Network Security Sweep the Disruptive Landscape?
In the past, companies used to depend on fixed, rule-based engines to monitor behavioural patterns for looming threats. And, the result was below-par. But today, with the developments in machine learning, organizations are authorized with data deception technology and live anomaly handling that leaves no trace scope for manual intervention and guesswork.
With the help of machine learning, security systems tailor-made for tracking threats can learn and analyze patterns to help avert similar attacks and counter changing behaviour. It’ll assist the security experts in being more energetic and dynamic when it comes to ruling out threats and countering live attacks.
You can come across various machine learning algorithms for network security like:
- Regression: Detects correlation between diverse datasets and perceives how they are linked to one other. You can use regression to figure out operating system calls and then track down anomalies by contrasting the prediction with the exact request.
- Clustering: Examines the datasets and sorts them based on the general characteristics of this tracked data. Clustering works with fresh data without considering the past examples.
- Classification: In this methodology, the algorithms are trained from the past information and try to employ the knowledge procured to the fresh data. The classification includes taking artifacts and arranging them under one unit of numerous labels. For instance, classify a binary file into several categories like adware, ransomware, or legitimate software.
If you strictly adhere to these guidelines, you can refine and restructure the overall effectiveness and productivity of your machine learning security administration. This is because they are inferred based on past decisions and behaviour patterns. In other words, it is a versatile system that’s highly potent when it comes to creating logical relationships hinged on available data. In addition, these tools can aid you with risk management and threat response.
- The synthesis of possibilities permits you to get fresh results following historical and new datasets. This strategy allows for an improved determination of the possibilities of repeating previous states of the system. For instance, synthesis can be employed to track down vulnerabilities in a company’s systems proactively.
- Forecasting is probably the most modern and advanced machine learning algorithm for data security processes. Possible results are derived by assessing the existing datasets. Forecasting is highly helpful when it comes to fraud prevention, threat modelling, and shielding the data getting violated.
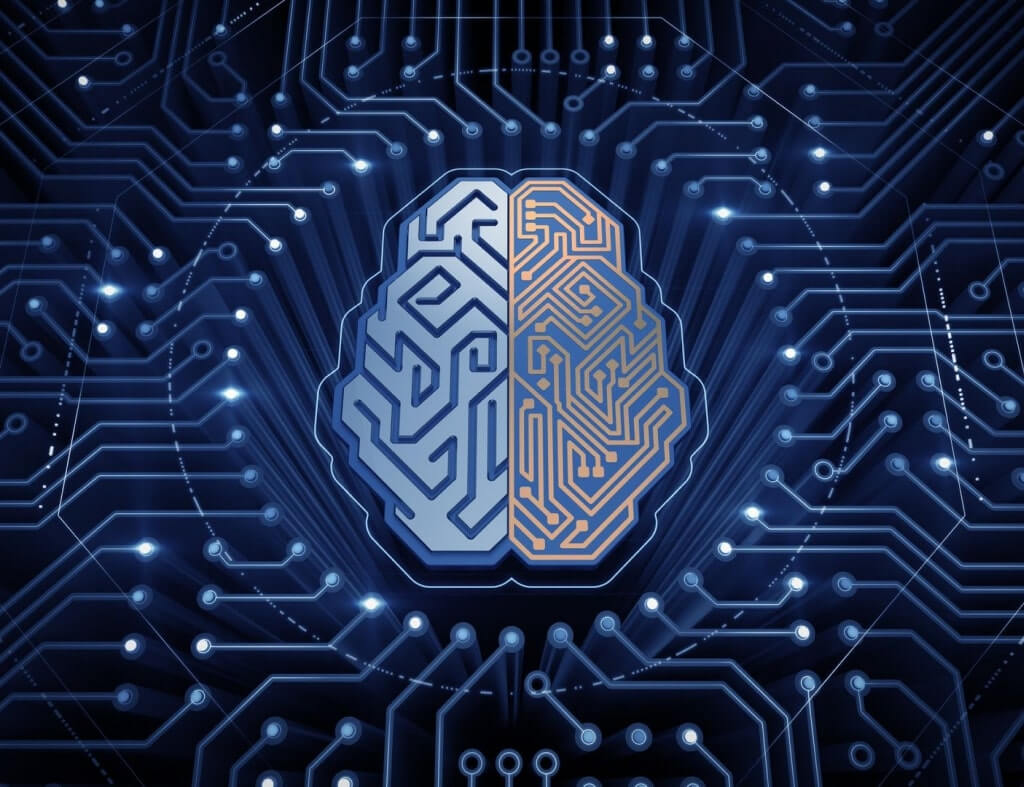
A reputable machine learning application development company knows that ML is the ideal security solution for today’s organizations looking to succeed. Security experts need robust assistance from intelligent machines and cutting-edge technologies like AI and ML for network security protection.
Benefits of Integrating Machine Learning in Information Security (InfoSec)
With artificial intelligence technologies like machine learning, analysts can rapidly respond to threats more confidently. Machine learning for security analytics permits an organization to swiftly view loads of data and examine them using statistics.
Today, a considerable amount of data crops up every day. So, it’s better to take the machine learning way and merge it with InfoSec to enable automation and precision and procure fruitful results.
- Processing of Huge Volumes of Data
A notable advantage of machine learning is its potential to process massive volumes of data since it automates the creation of algorithms to monitor security threats. The processed data covers a plethora of IT network components.
IT security and machine learning go side by side because this disruptive technology helps protect the shared files, emails, and several other information and tracks down the hacker activities and behaviour patterns.
With machine learning security analytics, one can process data in no time since it uses the artificial intelligence software that comes with top-notch processors. These processors assist in zipping through big data straightaway and drawing up solutions and anomalies to tackle these burning issues.
- Improved Vulnerability Management
Vulnerability management is the keystone when it comes to protecting an organization’s network. As stated earlier, organizations face security threats regularly and thus making it necessary to monitor, track down and prevent security breaches. Assessing and analyzing these existing security measures via machine learning can aid in vulnerability management.
Machine learning in computer security can help an organization assess systems quicker than regular cybersecurity personnel, strengthening your manifold problem-solving capabilities. In addition, it detects the weak links in computer systems and networks and helps organizations focus on crucial security tasks, helping them to secure systems on time.
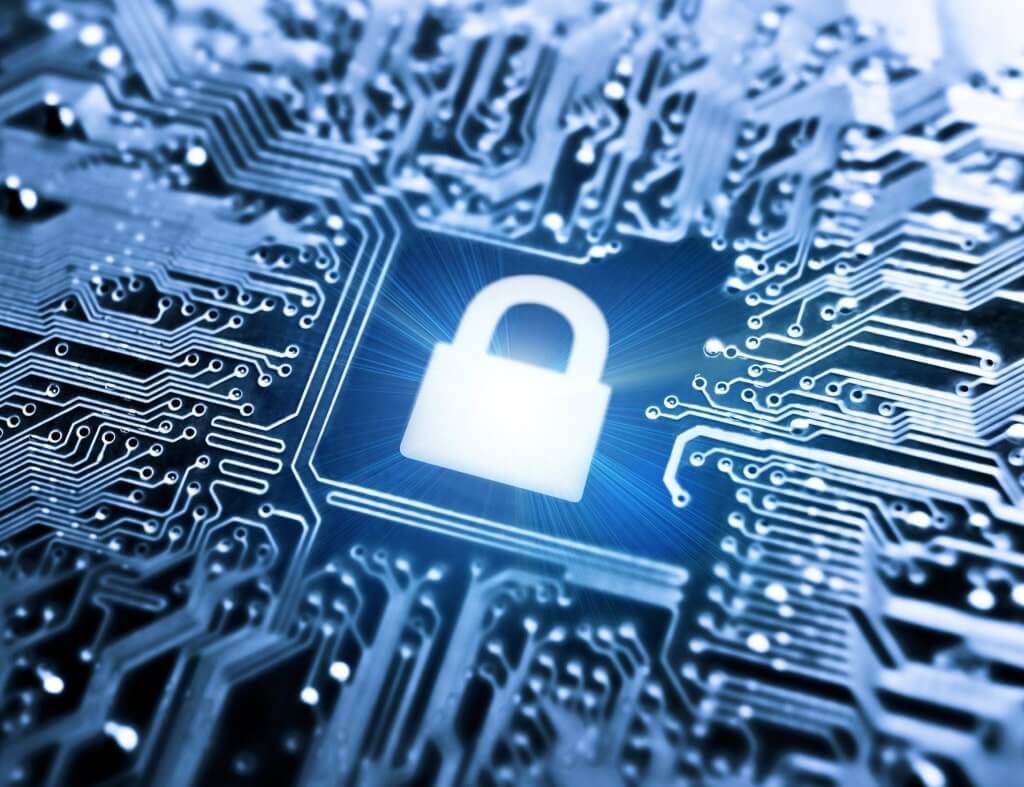
- Extracting the Slightest Threat in Cyber Haystacks
Cybercriminals always move stealthily, blending in the shadows to carry out attacks at the right moment. They also map out ways to infiltrate networks. Unfortunately, since they camouflage, these threats often go unnoticed, and an average cybersecurity expert fails to notice.
However, using machine learning algorithms for network security can help an organization to detect such security threats. It becomes easy to track and label even the littlest of moves and behaviour before entering the system, keeping organizations a step ahead of hackers.
- Shielding Cloud Data
Today, businesses migrate their databases to the cloud ecosystem to reduce maintenance hassle and external server loads. If companies embrace machine learning security analytics, they can protect data stored on the cloud platform. Machine learning helps to track down and label suspicious cloud logins and investigate IP addresses and their prominence.
- Analysis of Mobile Touch-Points
Machine learning is advancing to the world of mobile phones faster. The merger is fuelling a new age of information security. As a leading destination for AI app development services, we know that the future possibilities are unprecedented and endless when it comes to voice-based recognition experiences driven by Alexa, Google Assistant, or Siri.
Machine learning, fused with the Internet of Things (IoT) and AI, can gather data and harness the value to empower devices that perform on their own power and take instructed decisions, ensuring data protection.
- Tackling Diverse Hacking Techniques
There exist a plethora of advanced and complex hacking techniques that cybercriminals use to violate networks and data. For instance, polymorphism, obfuscation, etc. These hacking methods are challenging to track down, and the lack of top-level security personnel amplifies the issue. These threats can be worrisome because attackers can rapidly procure your personal information. Once the attackers get hold of your personal information, it blemishes your security systems.
By embracing and executing AI’s social honeypot and machine learning technologies, organizations can use it to entrap attackers. The honeypot system deflects attackers from a legitimate target. IT professionals set up decoys to identify the origins and hacking techniques used by cybercriminals to violate data.
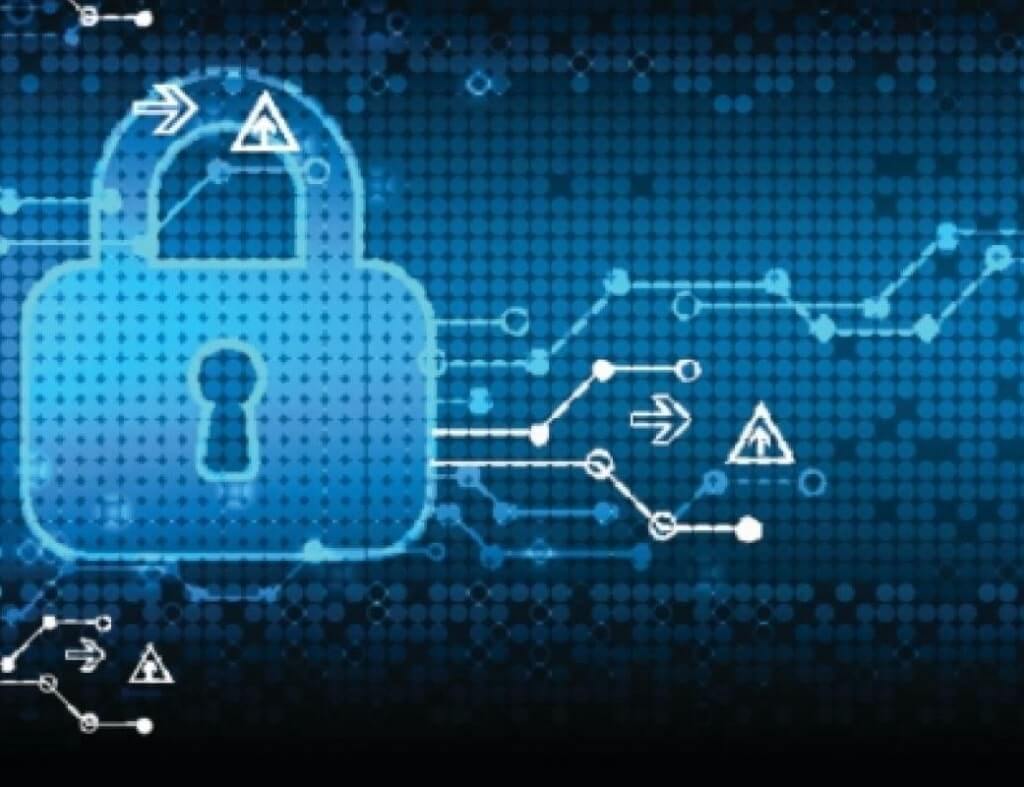
Future of Machine Learning for Network Security
Data security in machine learning is a powerful stimulus, hoisting a new programme of machine-powered humans and creating a best-in-class security landscape. Presently, the technology is prospering to help monitor and eliminate security threats. Henceforth, a dynamic and powerful level of differentiation will be accomplished when machine learning specialization becomes a part of breakthrough projects.
Machine learning algorithms for network security add an extra layer of protection against data violation. They extract essential information and patterns that help bolster proactive security systems. Therefore, businesses should lay out money in comprehensive and integrated InfoSec systems that drape all their setup ends in multi-layer machine learning-based models.
Final Words
Keeping your network and data safe is a tough nut to crack if looked at the present business ecosystem. However, you can take a groundbreaking step by embracing ML to bolster your overall security infrastructure.
AppStudio is a leading artificial intelligence development company with years of experience in data science app development. We provide end-to-end data security solutions highly customized, optimized, and constantly on guard, to ensure security from external threats. Contact us to learn more.
Frequently Asked Questions
Q1. How can machine learning impact InfoSec?
Machine learning plays a vital role in data security against external attacks as it can keep malicious attacks at bay.
Machine learning is an emerging trend assisting organizations in assessing digital attacks and eliminating them before its too late. By embracing machine learning, organizations can detect threats and counter malicious attacks. It also helps to automate arduous and burdensome tasks that security personnel carried out earlier.
Q2. Can machine learning be coupled with cybersecurity?
Yes, but it isn’t easy since it requires a great deal of understanding and knowledge of both realms.
Today, organizations face loads of sensitive data thievery, media manipulation, data breaching-related issues. The security experts and machine learning engineers can unite by creating a robust model that eliminates all sorts of data-related vulnerabilities and keeps the organization’s systems safe from external threats. If they’re able to develop this, it’d be the most groundbreaking crossover ever. Moreover, getting to train a self-sufficient model that defends itself from malicious attacks is itself a world-class innovation.
Q3. How can deep learning be used to deal with malware?
Instead of updating the firewalls with recently introduced threats by using a signature-based detection system, deep learning can come in handy to detect common attack patterns.
The traditional firewalls use a single-base detection system to detect malware. The company handling the database updates it with the recently introduced malware so that the firewall can detect it when that malware attacks the system. With a deep learning algorithm, one can detect advanced threats by learning the system, analyzing patterns, and recognizing suspicious activities that signal the presence of malware.